In the dynamic world of AI-Powered Customer Support, the integration of Large Language Models (LLMs) through platforms like Teneo is revolutionizing Conversational AI for Customer Service. The recent Galileo Hallucination Index, a benchmark for LLMs, highlights a crucial aspect of AI Call Center Technology – reducing LLM summarization hallucinations, a phenomenon where AI generates incorrect or fabricated text.
Benchmarking LLMs for Enhanced Customer Experience
The Galileo Hallucination Index is pivotal for enterprises implementing AI in Contact Centers, ensuring accuracy and reliability in customer interactions. This benchmark is a game-changer in Customer Experience Optimization and Automated Customer Service.
Benchmark for LLM Hallucinations
The Galileo Hallucination Index addresses a critical challenge in deploying generative AI: the risk of AI hallucinations in production environments, where the model generates incorrect or fabricated text. This index is crucial to reap the benefits of ai in customer service for businesses who are keen on implementing AI solutions without compromising the accuracy and reliability of the information provided.
LLM Hallucinations: The Power of OpenAI’s GPT-4 and Teneo’s RAG
OpenAI’s GPT-4 has shown remarkable performance in the Index when using Retrieval Augmented Generation (RAG), which is integral to Knowledge AI systems. However, the task type, dataset, and context significantly influence these results, underscoring the complexity of accurately measuring LLM hallucinations.
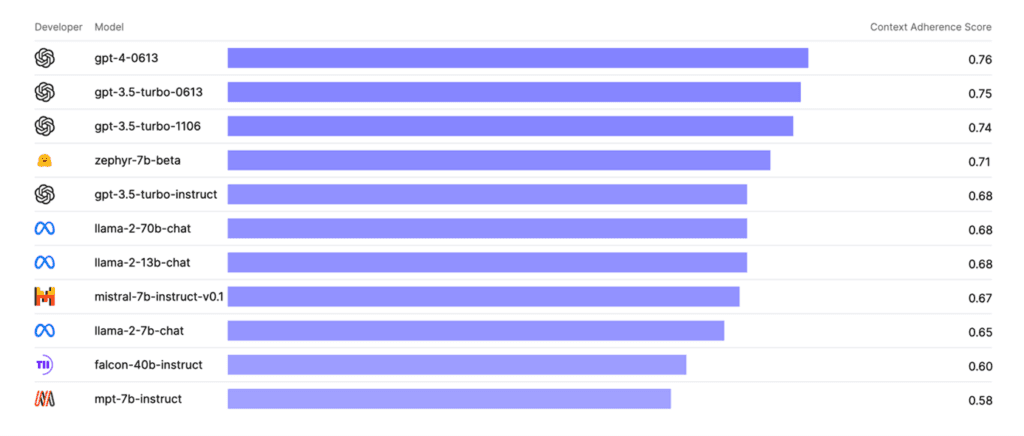
Model Comparisons and the Galileo Hallucination Index
The chart substantiates the importance of selecting the right model for the right task, as shown in the Galileo Hallucination Index. While OpenAI’s GPT-4 has demonstrated its prowess, it’s noteworthy that the more cost-effective and faster GPT-3.5 models offer competitive performance. Surprisingly, Huggingface’s Zephyr-7b, despite being significantly smaller than Meta’s Llama-2-70b, has outperformed its larger counterpart. This finding challenges the common assumption that larger models are inherently superior.
The lower end of the scale saw TII UAE’s Falcon-40b and Mosaic ML’s MPT-7b lagging behind, suggesting that size and architecture variations can critically influence the model’s performance.
The Importance of Benchmarking LLMs
The Galileo Hallucination Index underscores the importance of thorough benchmarking in understanding each model’s strengths and limitations. It guides enterprises toward informed decisions that align with their unique operational requirements and customer service goals in Generative AI.
Stay Ahead in AI Innovations – Subscribe to Our Newsletter
Subscribe to our newsletter
What is RAG?
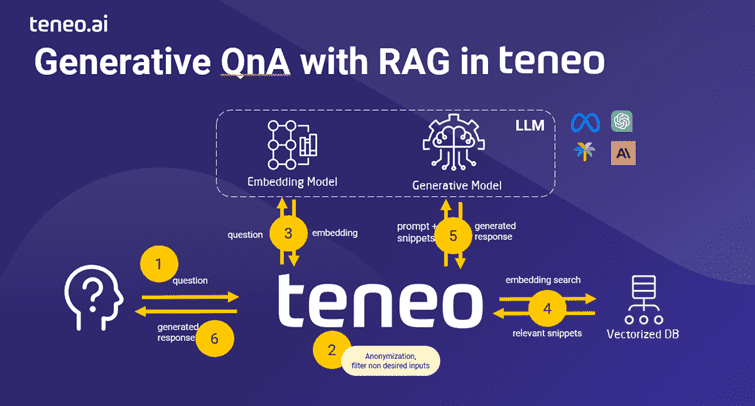
Retrieval-Augmented Generation (RAG) is a technique that enhances generative AI by merging the retrieval of information from databases with the generative capabilities of models like GPT. RAG first locates relevant information from a vast repository of data. Then, it leverages this context to generate coherent and precise responses. This is particularly vital in conversational AI. It allows the system to provide answers that are not only accurate but also deeply rooted in the specific content users are inquiring about.
Understanding Teneo’s RAG in Action
Teneo’s RAG is a key feature of our Knowledge AI. It delivers real-time, accurate answers by leveraging a vast repository of data, thus controlling hallucination in LLMs from both structured and unstructured data sources. Teneo’s approach ensures every customer interaction is accurate and consistent, raising the standard for customer service. Additionally, with Teneo’s advanced vector search technology and the ability to tailor any LLM to your needs, you can achieve unparalleled results and customer satisfaction in your Cloud Contact Center. Our approach ensures accuracy and consistency in every customer interaction, setting a new standard in Conversational AI for Customer Service.
Retrieval-Augmented Generation (RAG) in Teneo is a cornerstone of our AI in Contact Centers. It merges information retrieval with generative capabilities, ensuring Reducing Call Handling Time and enhancing Operational Efficiency in Contact Centers.
For Contact Center Automation, the platform also boosts agent performance. By providing interaction summaries and real-time response suggestions to the agents, it enhances the overall customer experience.
See Teneo’s RAG in Action: Request a Free Demo
Conquering Latency for Seamless AI Interactions
In the digital conversation landscape, ‘latency’ refers to the delay between a user’s request and the AI’s response. In real-time interactions, high latency can lead to awkward pauses that disrupt the natural flow of conversation, akin to speaking on a call with a bad connection. For AI-powered customer service solutions, reducing latency is not optional—it’s essential. Typically, a call to the Azure OpenAI API takes between 0.2-0.8 seconds for interactions with a relatively small prompt (e. g. the task instructions plus a user input like ‘My phone is broken’ which is being analyzed.
However, there can be outliers that take more than a second, potentially several seconds in the worst-case scenario. This latency can become problematic when multiple GPT calls are required within a single bot interaction. For example, when dealing with Sentiment Analysis, Queue Classification, and Entity Recognition. Reducing latency is crucial in AI-powered customer service solutions. Teneo’s recent update to its Teneo GPT Connector has improved multithreading for GPT, decreasing total latency by 147%.
This table shows latency data of five selected interactions with our Teneo, all using GPT-3.5 in milliseconds or seconds:
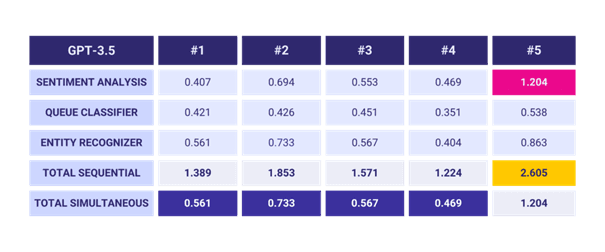
When GPT API calls are run sequentially, the response times of your bot can be just satisfactory, as seen in test cases #1 to #4. However, when these calls are executed simultaneously, the total latency time is reduced to the duration of the longest single call.
“To put it simply, by executing our GPT calls concurrently, we can decrease the total latency in our first example by 59.6%. Conversely, running the calls in a sequential setup can increase the latency by as much as 147.6%.”
Conclusion: Harnessing LLMs for Enhanced AI Communication
Navigating the landscape of conversational AI, we see the critical role of precise benchmarks like the Galileo Hallucination Index. Furthermore, the integration of sophisticated techniques like Teneo’s RAG, and the necessity of overcoming latency. The integration of sophisticated techniques like Teneo’s RAG, prompt attention to AI hallucination concerns, and the commitment to overcoming latency are the building blocks for robust AI-driven interactions. With LLMs, businesses are setting new standards in customer service excellence.
Have Questions About AI in Customer Service? Contact Our Experts!